2019 Winter Symposium
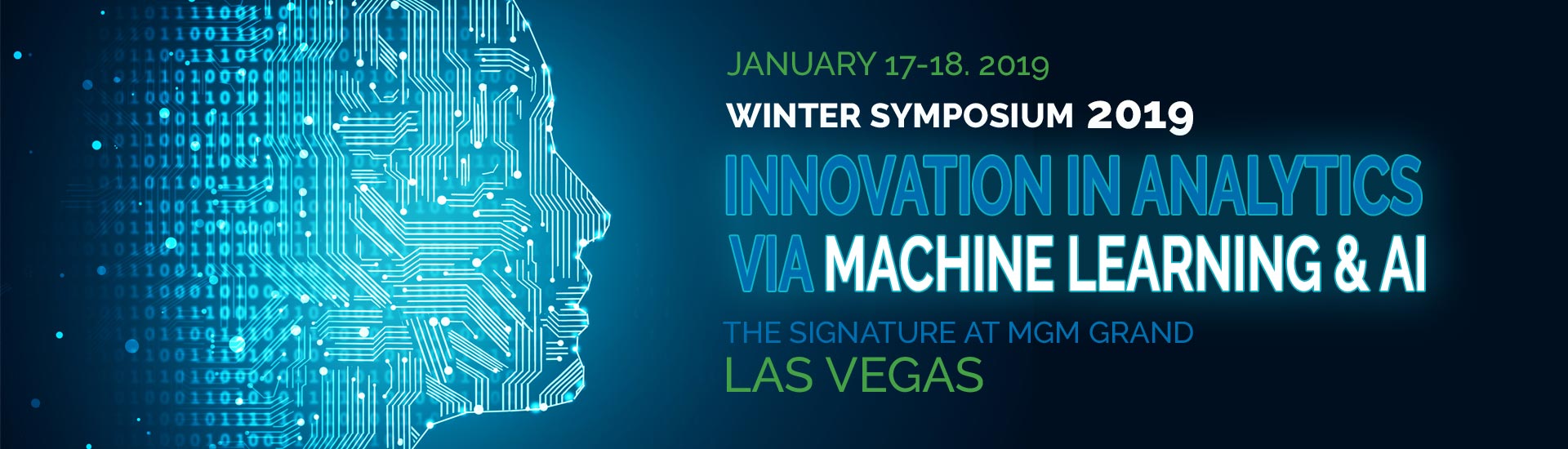
Artificial intelligence and machine learning are being hailed as the future of data analytics. Computer models can analyze high volumes of real-time data to make more accurate market predictions. How are these powerful tools currently being leveraged by pharma data scientists, and what does the future hold for AI in the pharma industry?
THURSDAY, JANUARY 17, 2019 |
|
---|---|
07:00 AM - 08:00 AM |
Breakfast |
08:00 AM - 09:30 AM |
Patients traverse a complex journey for a disease within the healthcare system. Certain diseases are simple to identify, such as diabetes, hypertension, or atherosclerosis. These conditions can be definitively diagnosed via a series of lab or imaging tests. However, patients with medical conditions such as multiple sclerosis, fatty liver disease, and rare genetic disorders may pass through multiple healthcare providers with symptoms, series of lab and imaging tests, and multiple misdiagnoses along their journey. Portions of of the journey are well understood, while some of the patient characteristics and related treatment behavior are not so obvious. Recently, machine learning classification algorithms have been used to find patients with a high propensity for a diagnosis/treatment by analyzing events in the patient’s history. While these algorithms have worked very well, looking under the hood can be challenging. This workshop addresses novel approaches to “decipher” the complexities of the black box and discover latent insights along the patient journey to help find patients who are either likely to develop a disease or suitable for a particular treatment option. This can help healthcare providers understand the characteristics of these patients in order to arrive at earlier diagnosis and treatment, alleviating patient suffering and slowing or stopping disease progression. Speakers: Rajkumar Rajabathar, Engagement Manager, Symphony Health Solutions; Karin Hayes, Principal, Symphony Health Solutions; Viswa Balasubramanian, Vice President of Analytics & Architecture, Symphony Health Solutions; John Ridge, Executive Director, Sales Analytics and Operations, Retrophin |
09:30 AM - 09:45 AM |
Break |
09:45 AM - 11:15 AM |
Under-diagnosis poses a serious constraint on the size of the addressable market for many pharmaceutical products. Identifying rare disease patients and target physicians is challenging. Physicians’ often limited disease understanding, clinical variation and lack of screening capability in the health systems are a few of the factors that play into this challenge. Moreover, pharmaceutical organizations, have traditionally approached patient identification and physician targeting in isolation, resulting often in disjoint engagement strategies and thus unrealized opportunities to take the right drug to the right patient at the right time, capturing the anticipated addressable market. This presentation will demonstrate how novel artificial intelligence (AI)/machine learning (ML) methods drive commercial value by realising the true size of the addressable market by identifying undiagnosed patients and understanding physician behavior in parallel. Results will be illustrated across a handful of use-cases, including mostly rare but also common diseases demonstrating wide application of this AI/ML approach. Diagnostic accuracy will be compared to classical analytics methods demonstrating consistent outperformance. Results will be supported by a live demonstration of a novel AI/ML technology that enables deployment with quasi-real-time physician alerts based on refreshed data, capability for ROI monitoring and continuous algorithm feedback loop for patient and physician commercial strategy evaluation and adaptation. Speaker: Harietta Eleftherochorinou, Senior Principal, Machine Learning & AI Solutions, IQVIA |
11:15 AM - 12:15 PM |
What Makes an AI Strategy a Success? Pharma Case: NLP to Understand HCP Understanding the HCP and their relation toward a disease or a new drug is key to the pharmaceutical industry. A deep understanding of an HCP is indeed key for an efficient Marketing strategy and operation. Traditionally we entrust information on a topic that HCPs have provided themselves, through a survey, an interview, etc… The issue is that it is difficult to judge oneself. Ask anyone if they are interested in innovation, they will say yes. But are they all interested, and to the same degree? What if you could assess their relation with a specific topic in a more reliable manner? What if you could use their behavior toward medical content to do so? This presentation will go through the key factors for a successful AI strategy. We will use an AI using an NLP and Machine Learning to illustrate it, to answer the question above. Speaker: François-Xavier Rousselot, VP of Data Analytics and AI/ML Strategy, Aptus Health |
12:15 PM - 01:30 PM |
Lunch |
01:30 PM - 02:30 PM |
Leveraging Informed HCP Migration to Optimize Targeting and Multi-Channel Spend One of the biggest challenges facing launch brands in unique therapeutic areas is identifying the right target audience for unbranded and ultimately branded messaging. While some treatment options may be more intuitive than others, it is still a critical consideration that can impact speed to market, awareness and trial, business impact and ROI. Typically, early stage engagement becomes a surrogate for performance, and is a critical first step in defining and predicting the migration of HCPs along the prescribing continuum from awareness and trial to adoption and loyalty. Identifying these audiences, and analysing their message engagement in the early stages of a campaign, accommodates “in -program” adjustment of messaging and channels. This research will demonstrate the migration of HCPs from initial touch to progressively increasing levels of engagement leading to defined levels of Rx activity. Migration patterns are quantified based on writing activity for each period. This approach provides early insight into binary segmentation of HCPs into bio-naïve vs innovators. It also identifies the content and sequence that produces trial, and migrates HCPs from the primary levels of engagement to higher quintiles of Rx activity. In an environment where all eyes are on machine learning and AI driven algorithms to predict next best action, this work reinforces the role of strategy, marketing and analytics working together to test, design and leverage real-time insight. Furthermore, it validates and reinforces target selection, communication messaging, sequence, and activity base segmentation. Speakers: Kent Groves, PhD, VP Global Health Strategy, Merkle Health; Olympia Mantsios, Associate Director, Analytics, Merkle Health |
02:30 PM - 03:30 PM |
Patient Co-pay Card Optimization: An Integrated Approach Leveraging Machine Learning/AI Patient co-pay programs are widely used today as a tool to help drive patient conversion and adherence by decreasing patients’ out-of-pocket prescription costs. While co-pay cards make the brands prescribed by their doctors more affordable for the patients and thus help them be more compliant, co-pay program costs can make up a substantial portion of the brand’s marketing budget. In this presentation, we will share an analytic solution using machine learning/AI algorithms to design a more efficient co-pay program that will reduce cost for the brands without a negative impact on patient adherence. The overall solution approaches the problem from both the patient side and the HCP side. First, it helps identify the appropriate level of financial support to patients by better matching patient attributes with co-pay program offerings. Second, it provides intelligence to better target HCPs to improve co-pay kit distribution and utilization. Finally, we will illustrate how we can use these algorithms within an AI decision engine leveraging the most up-to-date information to implement a fully optimized copay card program. We will take the audience through the whole solution with a case study. Speakers: John Lin, SVP, Practice Lead, Strategy & Insights – Analytic Sciences, Epsilon Health; Eleanor Tipa, Vice President, Analytic Sciences, Epsilon Health |
03:30 PM - 03:45 PM |
Break |
03:45 PM - 05:00 PM |
Panel Discussion: Conceptualizing Data Science Capabilities and Organizational Structure Within Pharma Manufacturers Moderator: Scott Hull, US Advanced Analytics Lead, Shire Panelists: Ewa Kleczyk, Vice President, Client Analytics, Symphony Health; Mehul Shah, Director, Reporting & Analytics, Sanofi; Ashish Sharma, Principal, Axtria |
05:30 PM - 06:30 PM |
Reception |
FRIDAY, JANUARY 18, 2019 |
|
---|---|
07:00 AM - 08:00 AM |
Breakfast |
08:00 AM - 09:30 AM |
Machine Learning Applications in Commercial Life Sciences There are many complex business questions within the commercial life sciences industry that reside in an environment with numerous big data sources. These require rapid, comprehensive and repeatable solutions. Artificial Intelligence, which encompasses machine learning (ML), is rapidly being adopted as a panacea for solving such complex problems across industries. The power of ML lies in the ability to extract useful information from the data with minimal human intervention over time. This allows for the development and deployment of highly predictive models. In this talk, we will discuss the application of ML techniques on medical claims data. One of the important business problems is to gain an understanding of adoption patterns for a certain class of products. This problem can be split into two parts, which also allows us to demonstrate applications of unsupervised and supervised learning respectively.
Finally, we will discuss the current state of the art of ML applications in life sciences. We will review some of the latest work done and look at potential areas of future opportunity in the commercial life sciences industry. Speakers: Sravan Kumar Bhamidipati, Director, Decision Science, Axtria; Vijay Chickarmane, Manager, Decision Science, Axtria |
09:30 AM - 09:45 AM |
Break |
09:45 AM - 11:15 AM |
The two biggest problems are as follows. First, the predictive power of the Model is always lower than what we'd like it to be. The question is what can be done to boost the predictive power of the model and what the odds of success are. Second, the model does not explain its reasoning. It is up to us to understand why it does what it does. In other words, we are interpreting the model to provide an explanation. And here lies the problem: We draw the wrong conclusions. We'll discuss 3 ways to boost the predictive power of the model. The first one is to leverage domain expertise. This is essential but unfortunately may not take us very far. The second one is a more effective data acquisition strategy. It turns out that we tend to be more opportunistic than goal-driven. The third one, which is the road least traveled, is feature engineering. It the process of transforming variables into features, the lifeblood of the model. Neural Nets are notoriously black boxes. Explanation, if we can use this term, lies in the weights of the synapses that connect the neurons. Decision Trees, on the other hand, employ a representation that lends itself to interpretation. As for the other models, they lie somewhere in between. Unless we are attaching a dedicated explanation module to our predictive model, we are looking at interpretation rather than explanation. Here is the key problem: The data is not complete. Take for example a database that captures ice cream consumption and frequency of shark attacks. Say temperature is left out. We certainly will not infer that ice cream causes shark attacks and as a result we should ban eating ice cream. Yet, the same or a related mechanism may be at work with our data set. This is why for instance we were convinced until very recently that HRT drove down cardiac events when the opposite is true. We'll discuss how holes in the data lead the unsuspecting analysts to draw erroneous "insights" and profess bogus recommendations, and we'll describe a new kind of data asset that is very relevant for predictive modeling. This data source captures the dynamics of geographies at the state, county, and city levels. We'll explain why it is so relevant for predictive modeling and how it boosts the predictive power of our models. Speaker: JP Tsang, President, Bayser |
11:15 AM - 12:15 PM |
Machine Learning Against the Provider Graph DocGraph is the industry standard open data file showing shared patients in fee for service patient sharing patterns among providers in fee for service Medicare. It is the ultimate in real-world-evidence based “next best action” data. We have improved DocGraph graph data generation methods so that it no longer overestimates certain types of provider interactions. This new open dataset must be interpreted differently, and we will examine the caveats for applying machine learning methods to the graph for the purposes of affiliation modeling, patient journey mapping, KOL identification and other healthcare industry modeling purposes. Speaker: Fred Trotter, CTO, CareSet |
12:15 PM - 01:30 PM |
Lunch |
01:30 PM - 02:30 PM |
Driving adoption of new therapies requires engaging providers who are seeing the right patient, in the right care setting at the right time. However, most Next Best Action (NBA) models are powered only on provider behaviors, thereby under-representing the impact of patients’ choices on their care journey and outcomes. As an alternative to “Bring Your Own Data” (BYOD) Suggestions modules, we have built an NBA AI that can be used to drive timely and effective provider engagement out of the box. This AI platform is natively powered by Komodo’s “healthcare graph” of the United States, integrating 50 billion+ clinical encounters derived from 150+ payer complete datasets with client-supplied interactions to drive timely and accurate predictions. At the heart of this AI is the ability to scalably generate features across both patient cues and provider behaviors to accurately model conversion, accounting for local biases and sales rep performance. Our AI retrains weekly to avoid overfitting to historical data and inevitable shifts in what matters as products move through their lifecycle. In this discussion, we will share a pragmatic end-to-end NBA implementation built on a graph that consistently drove 10% higher monthly adoption rate without requiring significant infrastructure investment. Speaker: Arif Nathoo, CEO and Co-Founder, Komodo Health |
02:30 PM - 02:45 PM |
Break |
02:45 PM - 03:45 PM |
Application of NLP & PCA in Life Sciences & Healthcare The revolutionary moment of Machine Learning (ML) & Artificial Intelligence (AI) in Life Sciences & Healthcare has already begun. Our Data Science team is working on a wide variety of projects that are value driven with the intent to push them in production within months. Some of our innovative projects are focused on:
The intent of this presentation is to help event attendees gain some valuable insights into how complex AI techniques can be used in this industry and share details on the methodology that they can bring back to their organizations for immediate reference. The power of ML & AI will be presented by walking the audience through two business use cases:
For each of these topics, we will organize the presentation to address the following:
Speaker: Robin Varghese, Principal, CustomerInsights.AI |
03:45 PM - 04:00 PM |
Wrap Up |